Predicting, and Knowing What to Predict
(Note: This is adapted from a presentation a few weeks ago at the Manifest prediction markets conference. This NYT piece on the conference captures much of the flavor of the event.)
Every time History once again refuses to End, one of the meta questions it raises is: who predicted this? The prediction question is important because violence is the ultimate expression of uncertainty. You don't start a violent conflict if you're confident that you'll lose, and you don't need to start one if it's obvious that you'll win. Actually, one reason for the comparatively peaceful last half of the twentieth century was that the most relevant means of destruction had a quantifiable impact, and both sides were happy to share their exact position. Better information led to less conflict regardless of whether or not anyone wanted such a conflict. So, at least along this axis, a more certain future is a better one.
Predicting the future is easier with data, and there are two sources of information on what's coming next that provide real-time quantifiable estimates: traditional asset markets and prediction markets. They're both useful ways to keep track of geopolitical events, albeit imperfectly. Consider this:
That looks like a reasonable cadence of events: at the start of the year, the prediction was closely anchored to the low base rate for land wars in Europe, but over time, evidence accumulated and the prediction market moved closer to certainty.
But that screenshot is, sadly, a case of disinformation promulgated by alternative media outlets. It's actually a chart of front-month Brent crude futures:
And this illustrates some of the tradeoffs of using financial markets as prediction markets. For example, markets affect the outcomes they predict: the invasion calculation was more favorable to Russia when oil was expensive, since that would make sanctions less damaging and less enforceable ($). The fact that market prices can change fundamentals—a run in AI stocks, for example, will bring a lot more talent to AI companies and will encourage their suppliers to add GPU capacity, collect more data, etc.—means that they're always aiming imprecisely at a moving target. That kind of unstable system consisting of reactions-to-reactions is a feature of plenty of other multiplayer systems, from video games to politics. But it's frustrating to anyone who wants a precise answer.
Prediction markets are much, much better at giving people precise answers. They're also good at conditional answers: a hypothetically valuable set of prediction markets would have included questions like "Conditional on oil going to $x, what are the odds that Russia invades Ukraine in 2023." This would have illustrated the payoff function quite well.
But part of the trouble with prediction markets is that very precision. There really isn't much interest, especially for obscure issues; how much organic demand is there to punt on the question of which board member of Texas' energy grid will step down next? The other, subtler problem is that when someone does have an interest, it's usually because they have information. If someone starts betting heavily in this market, there's a tiny chance that they've suddenly developed a niche interest, and a very high chance that they have unique information. So a reasonable market-maker needs to quote a very wide market on niche topics. Or they need a market-making subsidy, an approach prediction market pioneer Robin Hanson has long advocated.
Uninformed traders are existing markets' answer to this subsidy problem. And these traders are "uninformed" in different ways and to varying degrees. Some of them are literally gambling, in a way that's random in the aggregate, but sometimes decidedly non-random, as during the meme stock era. It could also be a portfolio manager passively moving asset allocations around, or it could be the company itself, issuing stock because it needs to pay expenses, or mechanically buying back stock because it's a tax-efficient way to return capital to shareholders.[1] In short: if you're making a market in a liquid financial product, you can expect many of the traders you interact with to trade fairly randomly, and for few of them to have strong information about the immediate direction of the stock.
That combination results in low transaction costs, and that amounts to a subsidy for time and money spent gathering information. It's worth figuring out what will move shares of Apple or Nvidia 5%, or even 1%, because the cost of getting in and out is so low. For less liquid assets, the cost of trading essentially represents a tax on informed views. If shares of a company can be sold at $9.50 and bought at $10.50, you can correctly predict a 20% move but only break even after those transaction costs.[2]
A shortage of noise traders is not the only tricky issue. One nice thing about prediction markets is that they allow beliefs to be bet on at arbitrary levels of precision. One prediction market whose price moved a lot over the last few days was called "Will Nothing Ever Happen By Halloween?"[3] That market moved fast, of course. But in this case, there wasn't a ready market tracking the odds of Hamas launching an attack on Israel. It just wasn't much on the radar as a specific, salient risk.
But these markets will always be limited by the attention people pay to issues and the bets they make. It's hard to enumerate all of the Black Swans out there, even if that's what makes them Black Swans in the first place. This makes the tradition of making a big list of predictions every year a good Schelling Point for periodically thinking about topics that have low odds but might have higher odds than they used to. (Perhaps the thing to subsidize here is prediction markets on events that the average informed bettor might be expected to put 1% odds on, but where the real odds might be more like 5%.)
Financial markets give precise answers to a subset of questions that, while important to some of the participants, are generally not the big questions on anyone's mind. "What is the present value of the future cash flows generated by Nvidia?" To put it in prediction market and smart contract terms, they have a built in oracle in the form of the cash flows from stocks and bonds, and the delivery of commodities.
But that means they're intrinsically limited to the class of problems whose answers can be defined in cash flow terms: "what's the present value of future Tesla cash flows?" is a question that will ultimately be answered by those cash flows, whereas "is the cybertruck too ugly to succeed?" is not. This question gets answered implicitly and noisily by Tesla’s share price, but it’s hard to disaggregate the random moves in the stock from response to specific questions. So equity and bond markets end up being a decent cross-section of many parts of the economy, though they underweight businesses that are naturally fragmented or have low capital needs.[4] But they don't address most of the open questions.
They are adaptive, however; financial markets will quickly answer the question "what does this event change about the state of the world, as measured by cash flows from companies and the supply/demand balance of commodities and currency?" very quickly and generally efficiently. And that is a useful question to ask—if nothing else, it's a good way to do a quick Nothingburger Test on any given scary headline, by asking if the market reacted to it much.[5] For prediction markets to fill this function for the many, many specific questions for which an accurate answer is valuable, they need to cultivate a population of noise traders. Being well-informed pays in proportion to how many uninformed counterparties are willing to bet with you, so in the end gambling becomes a subsidy for certainty about the future.
And if we expand capital allocation to include money as well as time, we can even say that professional investors are a sort of noise trader: imagine one person who devotes 60-80 hours a week for their entire career to painstakingly tracking all the economic drivers that determine company performance in, say, the auto parts sector. All that effort ends up adding a basis point or two to the returns of some other investor who puts their money in index funds because they want to enjoy a relaxing retirement without worrying too much about how they'll pay for it. By the second trader's standard, the first one is valuation-insensitive with respect to their time, even though they're keenly aware of which company should trade at 6x EBITDA and which should be at 5.5x. ↩︎
Of course, you could use limit orders in this scenario, but in that case you're trading time for money: if your prediction comes true before you finish making the trade, you still lost. You’re also leaking information, and a market-maker might increase their bid in response. ↩︎
"Nothing Ever Happens" is a shorthand for the view that the long-term importance of most widely-discussed events is far overrated at the time. Sometimes, of course, Something Happens: it was a big deal when people in Wuhan started getting sick, but it would have been very easy to scroll past this AP story from January 7th, 2020 without a second thought. The Nothing Ever Happens hypothesis isn't that there are literally no world-altering events—after all, we live in a world that's been substantially altered from its starting conditions—but that you could probably trawl through news stories from the first week of January 2020 and find a hundred different topics that seemed at the time like a bigger deal. ↩︎
Yes, capital intensity is a trait of the cycle rather than just the industry, but there are some kinds of companies that have an attention bottleneck rather than a capital bottleneck, like consulting, law, accounting, and, oddly enough, proprietary trading. For these companies, there's no question that they can pay employees reasonable compensation, in cash, and thus don't need to capitalize their future profits, turn them into equity, and pay employees that way. These companies also generally don't want the distraction of preparing to go public and then answering to public shareholders. If they're private partnerships, their shareholder base is dominated by people who understand the business and like the company. Why add hedge funds to the mix? ↩︎
This is not infallible; crashes happen when markets underreact to risk for a long time and then overreact all at once. But it is good for addressing the credibility of claims about vast second-order impacts from specific headlines. ↩︎
A Word From Our Sponsors
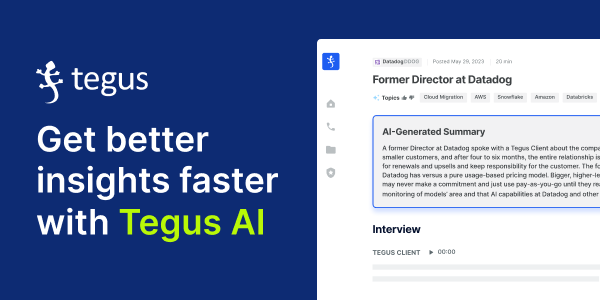
Manual search and data collection means time you’re NOT spending drawing insights, gaining perspective and making bold investment decisions. Let Tegus do the busy work for you.
Powerful AI and machine learning algorithms summarize each expert transcript to put key questions and themes front and center. And you get deep-dives into a single company, industry, or trend with auto-tagging across transcripts.Get better insights — faster — with Tegus’ latest innovations in AI.
Elsewhere
FTX Addenda
The Diff is generally not in the habit of providing ongoing coverage of big news stories, both because there's lots of competition and because the interesting questions tend to arise either on day one or months to years after the fact. But sometimes there's enough ongoing news flow to justify continued coverage.
This section of the testimony helps explain one of the big FTX questions: where did the money go? I've handwaved this a bit, because obviously FTX ran out of money and clearly Alameda had access to FTX's money and spent a lot of it. But one lingering question is how the gap got as big as it did: I'd wondered if FTX was continuously borrowing to expand its directional trades, borrowing against directional trades to fund all of its market-making, or something else. But one answer is that in cases where an FTX customer lost more than 100% of their equity, Alameda was taking the loss.
Part of the original pitch for FTX was that when exchanges offered levered products, sometimes customers on one side of a trade got wiped out. If you're long a 20:1 Doge futures contract and Doge goes up 10%, you've tripled your money, but the seller's loss is twice their initial equity. The old system was that some of your profits would be clawed back. But if Alameda steps in and retroactively buys all the Doge down 5%, you don't get clawed back. But now Alameda's balance can go negative! The net effect of all of this is that an exchange that offers futures and does its own clearing can, indeed, experience a run on the bank, and not necessarily one that coincides with customer losses. Instead, it can bleed a little liquidity every time it takes on a bad trade.
This raises more follow-up questions, though:
- If competing exchanges were clawing back profits when traders blew up, why didn't FTX just offer a level of leverage that made this avoidable? One answer is that all of the insurance fund stuff was for show, and that they really expected to run an exchange that had negative profits once it ate losses from large market swings. Another possibility is that they honestly thought this was the right business decision: they'd attract volume with high-leverage contracts that appealed to small speculators, but eventually get dominant market share with customers of all sizes, at which point the crypto market would function more smoothly and these blowups wouldn't happen. (A decent fraction of accounting frauds start with a one-time effort to delay recognizing a loss, but this snowballs over time: target a higher level of return than you can earn with a given level of risk, or a lower amount of risk than is required to hit your target return, and you're fundamentally running a martingale strategy that will eventually blow up.)
- Will winning traders get their profits clawed back? One story from the trial is that someone exploited a bug in FTX's margin calculation, and took a huge position in Mobilecoin, and that Alameda ended up making good on the losses when this counterparty blew up. If the traders who bet against Mobilecoin made money, and they withdrew some of those profits, then the money they withdrew really belonged to other FTX customers: the insurance fund was wiped out, and the standard procedure for other exchanges was that the winning side of the trade wouldn't collect all of their winnings. FTX's lackadaisical attitude towards corporate records means that there's a chance these people won't ever be tracked down, but they are the (presumably accidental) recipients of the proceeds of fraud.
Slack and M&A Integration
Slack employees are being strongly encouraged to take time off their regular duties in order to use a training tool built by their parent company, Salesforce. This story has lots of the classic grumbles about mandatory corporate training ("Two sources told Fortune that many Slack engineers have found workarounds via scripts, code that can be quickly built to automate a task, to click through the modules for them"). But it's also an interesting statement about how hard it is to integrate acquisitions with a parent company. If Slack would run the same way inside Salesforce as out of it, M&A isn't really necessary; it can't be easier and cheaper to buy the entire company than to come up with a reasonable split for some kind of long-term cross-selling deal. So the newly-acquired company needs to be run differently than when it was independent in order to justify the purchase. But that limits acquirers based on the culture fit of what they're buying, or their willingness to lose employees who wanted to work at a company like Slack rather than a company like Salesforce.
The Global Meme Stock Rally
Financial trends in one country sometimes have an echo boom in a different market, because the same thesis or phenomenon can be applied to local circumstances; about two years after the wild bull market in US Internet stocks ended, there was a relatively larger one in the handful of US-traded Chinese Internet companies. (NetEase rose 38x from the beginning of 2002 to the end of 2003, and while Sina and Sohu are no longer listed, they also had extremely strong performance in this period.) It's been a few years since meme stocks were the dominant US financial headline, but now small-cap stocks, especially shares of highly levered companies, are outperforming in India, partly driven by investor discussions in WhatsApp groups ($, FT). Compared to Reddit, WhatsApp doesn't have nearly the same level of public visibility; hedge funds could and did (and do) monitor chatter on Reddit and Twitter to see if retail investors are getting excited about a highly-shorted stock. If the discussions are in smaller groups that aren't necessarily open, that could crimp virality a bit but reduces legibility more. And, of course, if a stock is being promoted on an app that's also used for urgent communications, the spread can be every bit as viral, especially if by the time a recipient gets the message, the stock is already moving.
Shrinkage
Home Depot tracked some retail thefts to a fence who was running an addiction recovery center ($, WSJ). One hypothesis on shrinkage is that it's gotten more systematic as it's gotten easier to sell stolen goods online. Marketplaces that allow third-party merchants represent an easy venue for selling some goods, and that ends up encouraging the regular theft of the same high-margin products. But what it also does is create a central node in the network that's easier to track and disrupt. As in many legitimate businesses, any operation that scales fast by relying on someone else's network can see their business collapse just as fast when the host network changes the incentives.
The Coasian Border in Ridesharing
A small ridesharing company, Alto, competes with Uber and Lyft by providing employees with regular schedules and flat hourly rates. This is a move some of the larger ridesharing companies have made when it's legally mandated, and it's interesting to look at what incentive changes are downstream from this:
- The writer of the piece takes a ride, and at one point the driver (using Alto's maps) goes in the wrong direction. "For an Uber driver, it would have been a major setback, potentially altering the amount of money the trip would pay. But Mr. Arndt just shrugged — it was no problem — and began navigating toward downtown."
- The cost of a trip from the airport is about double what Uber charges.
- Alto offers a 30% discount for people who pay a monthly subscription (Uber's membership offers an unspecified discount on rides, but it's probably not 30%).
- Alto provides vehicles and uniforms for drivers instead of asking them to bring their own.
So the shift to a model closer to a scheduled service ends up reducing driver utilization, and ultimately utilization is what drives revenue per hour, which sets a maximum long-term wage for drivers. The gig worker model has many annoyances, but it tends to offer the lowest price for passengers and to be the high bidder for drivers.
Diff Jobs
Companies in the Diff network are actively looking for talent. A sampling of current open roles:
- A well funded seed stage startup founded by former SpaceX engineers is building software tools for hardware engineering. They're looking for a full stack engineer interested in developing highly scalable mission-critical tools for satellites, rockets, and other complex machines. (Los Angeles)
- A company reinventing the way Americans build wealth for the long-run by enabling them to access "Universal Basic Capital" is looking for a GTM / growth lead. (NYC)
- A data consultancy is looking for a senior data scientist with prior experience in marketing data science and e-commerce. (NYC)
- A startup building a new financial market within a multi-trillion dollar asset class is looking for generalists with banking and legal experience. (US, Remote)
- A crypto proprietary trading firm is actively seeking systematic-oriented traders with crypto experience—ideally someone with experience across a variety of exchanges and tokens. (Remote)
Even if you don't see an exact match for your skills and interests right now, we're happy to talk early so we can let you know if a good opportunity comes up.
If you’re at a company that's looking for talent, we should talk! Diff Jobs works with companies across fintech, hard tech, consumer software, enterprise software, and other areas—any company where finding unusually effective people is a top priority.