I'm doing a Callin interview with author Jimmy Soni today at 4pm ET/1pm PT. He and I will be talking about The Founders, his new book about the early days of PayPal. I highly recommend reading this—there's a lot that I didn't know, including both fun details and broad context.
Facial Recognition as a Pareto Technology
Facial recognition is a fraught topic: it can lead to false accusations, false convictions, and other injustices; it's hard to audit, since it relies on "black box" algorithms that are only as good as the data they're fed—at best. And it can be used in a targeted way, with little to no oversight.
But enough about the limitations of eyewitness testimony. Let's talk about automated facial recognition instead.
Automated facial recognition is an important technology to watch for several reasons:
- It's coming, and the choice is not "will it exist or won't it?" but "Which countries will have it, and how will they use it?"
- Like a number of new technologies, it does things that humans already do, with a different level of accuracy and much more extreme scale.
- Big tech companies have largely withdrawn from the market; Amazon set a moratorium on law enforcement use of facial recognition, as did Microsoft, and and Meta got out of the business entirely and deleted their data. When a new product is being built by entirely new companies, with much less big tech competition, the incentives are cleaner but predicting their behavior gets harder.
In one sense, software achieving parity with humans is a strict net gain: we're getting the same outputs with less input. But the economy is full of load-bearing inefficiencies. For example, consider taxes on tips: in theory, tips are taxable income and should be carefully documented and reported to the relevant tax authorities. In many establishments, they are. In others, they are not; there's a tacit social contract that tip income is mostly untaxed, and that it really doesn't do much good for the world to make people living below the poverty line make slightly higher FICA contributions. In other political debates, the impracticality of enforcing one side's agenda is what keeps things civil: you can sustain an indefinite stalemate between the unpleasant status quo and an alternative that is viewed by some people as utopian, by others as dystopian, and by every smart person as impossible.
This means that technology is inherently politically destabilizing; it breaks truces by changing what's possible. In China, facial recognition fits in nicely with the government's model, where surveillance is essentially a source of legitimacy. So it makes sense that one of the bigger facial recognition companies, SenseTIme, is now public and has a $39bn market cap. (I wrote about it when the prospectus came out here ($).)
SenseTime's product is impressive; from what I've heard about demos, users can select a single person from CCTV footage and follow them, or backtrack and see where they've gone. This is naturally helpful in maintaining one of the CCP's current sources of legitimacy—Zero Covid is much easier when contact tracing can be done without asking anyone or relying on potentially faulty memories. But it's also an effective tool for other parts of the model; dissident networks can't easily thrive online because network analysis is so easy, but it's hard for them to function in the real world if the same kind of network analysis can use camera footage, too.
So that's one model of what AI-based facial recognition is for: automating the labor-intensive parts of an intrusive state, in order to make it more intrusive.
There is another model, which one could call Pareto Technology: a Pareto improvement is any change in the status quo that makes some people better off and nobody worse off.1 Pareto Technologies can be considered technologies whose adoption makes some existing processes more efficient without expanding the scope of what's being done, in order to contain the unforeseen second-order consequences. It's hard for EVs to be a Pareto technology; the network effects of charging and the scale economics of batteries mean that their growth tends to be self-accelerating. But higher-mileage cars do look like a Pareto technology: they either mean the same trips take less fuel or that there are new opportunities for travel thanks to cheaper fuel.
For facial recognition, a product so fraught that the only entities that feel perfectly safe using it are governments, Pareto Technology status can be imposed more or less by fiat. So Clearview AI, a facial recognition provider has a complicated set of usage rules for its product—it's only available to law enforcement, all searches have audit lots, every search has to start with a case number and crime type, and there are no percentage scores estimating the probability of a match.
What most of these do is, if not eliminate, at least create significant roadblocks for abusive use of facial recognition; it's hard to cyberstalk someone if there's going to be an indelible record of it and the stalker will have to explain why he suspected his ex was involved in a bank robbery or whatever. The percentage scores piece is a UX decision worth dwelling on, both for what it says about new facial recognition technology and what it says about the status quo.
Humans are notoriously bad at a) dealing with probabilities, and b) converting subjective statements about probability into actual odds. This famous survey is one of the better illustrations:
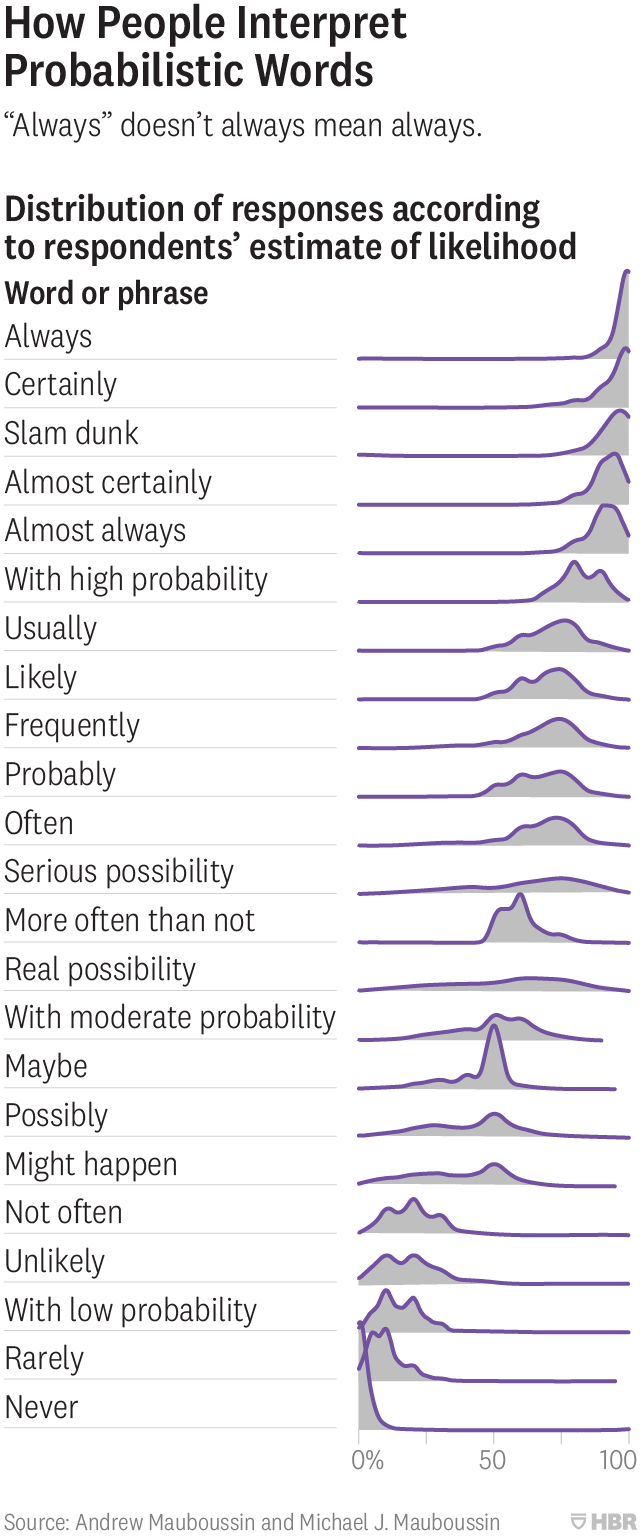
That's especially hard when you're interpreting the probability that a photo matches a specific person. Does "99%" mean that there's a 99% chance that you have the right person and a 1% chance you don't? Does it mean that 99% of people aren't a match for the photo, so the person is one of 3.3 million possible matches in the US? And given the fuzzy nature of other kinds of evidence, how do quantifiable odds compare to more subjective but meaningful indicators of guilt or innocence?
A more rigorous probabilistic approach would do the justice system a lot of good; courts are often insufficiently Bayesian, which can lead unlucky people to get sentenced based on bad math (here's another example), not to mention some truly eye-popping jury awards.
But solving that problem partially means making it worse; it means creating overconfidence in odds that are explicitly quantified and relative underconfidence in everything else. So the Pareto Technologist is forced to remove a feature that offers additional information; that information is hard to interpret and easy to misuse.
For any kind of technology that gives law enforcement better information, there's the usual who-watches-the-watchmen question: how do we know this isn't being misused? Oddly, a different technological trend takes care of part of this: facial recognition services tend to be cloud-based rather than on-prem. Partly that's because they're new and data-intensive services, and because it doesn't make sense for a local government's IT department to be a single point of failure for law enforcement. But cloud-based services also make it easier to log things, and to ensure that top-level policies are enforced everywhere; if every user action requires communication with a central system, it's easy for that system to proactively notice and respond to suspicious activity.
But that cloud basis is closer to leverage, not a strict improvement: it does mean that the US can set up meaningful guardrails around facial recognition use, and expect them to be enforced. But it also means that facial recognition is controlled by a company in one country, the way it's used is, to some extent, up to that country; perhaps when SenseTime is used abroad and is asked to identify, say, personnel at the Chinese embassy, it sometimes draws a blank. Governments are naturally entitled to be careful about who they sell strategically important technologies to, and how those technologies are used. Equally, though, buyers are entitled to consider which vendor is going to demand which terms.
Late last year the National Institute of Standards and Technology ran a test of facial recognition vendors' software, which put SenseTime at the top of the rankings and Clearview close behind. So choosing a vendor globally means either choosing China or the US. Clearview is not without its controversies. For one thing, the company collected data by scraping photos on sites like Facebook, which has led to all sorts of legal entanglements. There are other ways to get photos; some facial recognition vendors use mugshot photos, for example. This has the benefit of being more legally straightforward, easier to scale, and less likely to make people feel like their privacy has been violated.2 It has two enormous drawbacks: first, it's a small sample size, meaning that there's limited accuracy. And second, what it's accurate at doing is identifying people who either a) have been arrested before, or b) happen to look like someone who has been arrested before according to a model working with limited data. It's easy to see where this approach can go wrong. And then there's SenseTime, which does a lot of business with a government that mandated facial recognition for all smartphone users a few years ago. This is also a way to get lots of well-labeled training data.
If facial recognition is used as a replacement for other kinds of evidence-gathering, then it opens up all sorts of questions that are very hard to answer. If it's used as part of the investigative process, but only as a way to gather other kinds of evidence that are then used in a courtroom, it's very useful—it replaces general descriptions of suspects with a name and a most recent address, for example. And it's especially useful for investigating sexual abuse imagery, in which the perpetrators can be visible. This is a category of crime the US legal system takes incredibly seriously, and on its own basically ensures that there will be some form of facial recognition technology used for law enforcement. The algorithm is never enough to convict someone, and shouldn't be, but it can be enough to narrow down the search space—which means simultaneously increasing the chances of solving the crime and reducing the odds that innocent people get swept up in an investigation.
Can the Pareto Technology approach work? One example, which I've linked to before, is that various subgroups among the Amish adopt different technologies depending on which they think will be less disruptive. Doing this with everything is a bit extreme, but matters of law enforcement are also questions about the tradeoffs between rights, which are intrinsically a fraught question. Whatever arrangements we have are basically satisfactory in part because of technological limits, so every new development creates potentially worse outcomes. But the fundamental nature of technological advancement is that it means getting more from less. If that's inevitable, the social question is to figure out: more of what, and less of what else?
Thanks to Hoan Ton-That, cofounder and CEO of Clearview AI, for talking with me about the company's view of facial recognition.
Disclosure: I own shares of Amazon and Microsoft.
Diff Jobs
Diff Jobs is our recruiting service associated with the newsletter: we introduce Diff readers to companies who are looking to hire them. This is structured as a classic contingency recruiting service; no cost at all to candidates, and a commission charged to companies only once there's a placement. We're working with a variety of firms across fintech, proptech, healthcare, logistics, education, e-commerce, and some companies doing something so new there isn't a name for their category yet.
Some of our current active roles:
- A fintech company is looking for Data Engineers and Data Analysts to help institutional investors manage risk. (US, remote)
- A seed stage company is looking for backend engineers who want to help small businesses improve their margins. (US, remote)
- A startup building a tool to make the software development process easier, is looking for people who have the interpersonal skills to help teams implement the product, and the technical skills to facilitate this. (East Coast or London)
- A startup is building a tool to help small businesses untangle their vendor relationships and make better choices about what products they buy. They're looking for a BizOps generalist, which could be the right role for someone looking to transition from finance to tech. (US, remote)
- A startup broadening financial access in emerging markets is looking for a Senior Product Manager. (Brazil or NYC, remote in EST; fluent in Portuguese a big plus)
- A series B startup in the edtech space is looking for a Senior Product Designer. (US, remote)
Elsewhere
Aligned Incentives
I've written a few times (like here ($)) about Amazon's increasingly common policy of buying warrants in its suppliers. This has the obvious benefit to Amazon that if dealing with Amazon turns out to be profitable, or is perceived that way, then Amazon will still capture some of the upside. And a theoretical benefit to the supplier is that Amazon has a slightly stronger incentive to stick with them; saving a little bit by taking the low bid doesn't make sense if it comes with a giant capital loss. Not always, though; Amazon supplier and investee Rivian has lost about 10% of its market cap in the last two days on the news that Amazon is working with another supplier. Amazon owned about 160m shares at the IPO, so whatever their savings were on buying EVs from Stellantis and selling them AWS, those have to be compared to the cost of $1.6bn in losses on Rivian stock.
Lord, Make My Country Low-Emissions—But Not Yet
You can calculate the average carbon output of various fuels, but that's an average that doesn't tell you much about the marginal impact. All oil and gas extraction requires energy, and some gets a high energy return on investment (like continuing to pump from Ghawar) while others are lower (Lessons from the Titans points out that in 2012, before fracking surpassed conventional as the main source of oil and gas, it had already exceeded it in terms of horsepower used). One of the lowest has been oil sands. Canada has defended this even though it makes it harder for Canada to achieve emissions goals ($, FT): "For the [oil] demand that continues to exist, Canada needs to extract value from its resources, just like the United States, the United Kingdom in the North Sea, and Norway." It's easy to set long-term goals, especially if the hard decisions required to achieve them will be someone else's problem.
Elasticity
Kazakhstan is going through some significant unrest right now; a dozen police and many more protestors have died in recent days. The country produces about 39% of the world's uranium and 2% of the world's oil. In response to the disruption, oil prices are up about 3%. and uranium prices are up around 10% ($, WSJ). One of the reasons oil gets so much attention is that it's volatile, and one driver of that volatility is that global oil storage capacity is under 20% of annual production, whereas the world has a few years of uranium stockpiles. So temporary disruptions in the uranium market don't lead to panic-buying—there is enough to go around, and governments don't want the lights to go off in France or the US because of unrest in Central Asia. And that abundant backup supply means that the current price can also react to the long-term outlook: if uranium supplies do get threatened over long periods, that affects the decision to build nuclear power plants.
Google/TikTok Coopetition
Shopify's SEO lead writes about why Google wanted to let TikTok become the world's most-visited website. The basic argument: highlighting TikTok videos in search results was 1) good for users, 2) a good way to stay safe from antitrust, and 3) a way for Google to gather data on TikTok videos' popularity, so that if they decide that antitrust is less of a concern they can try to build a short video franchise through YouTube. It's a good look at the complexities of company relationships. Sometimes the best way to compete with a company in the future is to cooperate right now.
The Remittance Economy
I wrote in 2020 about how remittances were keeping Mexico's heavily oil- and tourism-dependent economy afloat early in the pandemic ($), and before that about remittances as a sovereign wealth fund. Mexico essentially has an equity-like claim on a share of US economic growth, making the country's economy hedged in cases where emerging markets are weak but the US is relatively strong. This is now something President AMLO acknowledges, saying that remittance growth is the reason Mexico has avoided an economic crisis.
If you want to dive into this you can always find ways to debate specific allegedly Pareto-optimal scenarios. For example, one seemingly Pareto-optimal policy is to simultaneously reduce trade barriers and increase transfer payments; factory workers may lose their jobs, but if the overall economy is bigger then there's enough money to make them financially better-off. But this implicitly assumes that a UBI check is just as good as a wage (could be better, could be worse, depends on who you ask and when). So, I'd think of Pareto optimality as the spirit of a solution rather than a 100% literal description of it; there can always be hypothetical edge cases, but the general idea is to design a future distribution that maintains the status quo except where it can be improved.
Economics has a long history of starting with practical questions like "how do nails get manufactured, anyway" and ending up thinking about really deep questions like the intrinsic value of human labor, how well utilitarianism captures human values, and the limits to knowledge and hubris. Adam Smith was writing about the nature of the good life before he started arguing about tariffs. ↩
The question of the degree to which this is a privacy violation is an interesting and probably endless one. On the one hand, you have the obvious point that a stranger can tell who you are by looking at a photo, although right now that stranger needs to be a police officer investigating a crime. On the other hand, the data was already out there; Clearview scraped things that were public, and put them in a different form. This somewhat mitigates it, and makes it closer to getting in trouble in the real world for something you tweeted. On the other other hand, people don't create Facebook photos in order to make it more convenient for them to get arrested. This is the kind of question that will get hashed out between privacy advocates and law enforcement, perhaps with an assist from the sites getting scraped. It's an issue the facial recognition companies themselves probably want other parties to solve. ↩