In this issue:
- Wish: E-Commerce, Fast and Slow
- Online-to-Offline
- <$10/month
- Movie Lots
- Taxes and Incentives
- Unfairness
Wish: E-Commerce, Fast and Slow
From time to time, but more rarely in the last year or two, there’s been a cogent bear case about Facebook: it turns out a huge chunk of their ad revenue comes from just one company, or just one category. The exact company varies—early on, it was the daily deal operators (who relentlessly copied one another’s ad copy) and the gaming companies (Zynga alone was 12% of Facebook’s revenue the year before the IPO). More recently, the worries have centered around Wish.
This turns out to be a problem that partly solves itself. Companies only end up being a large share of Facebook when their unit economics support lots of expensive direct-response ads. But this dynamic creates a deep order book for any given Facebook ad. When one advertiser drops out, there are many more who barely lost the auction for a given user’s attention.
Wish, whose parent company ContextLogic filed its S-1 last week, is a great case study in how these economics work out for advertisers, and is interesting on its own as a case study in revitalizing an old business model, and undercutting some key e-commerce assumptions.
Wish’s main product is an e-commerce app geared towards consumers who are sensitive to price and little else. Browsing the app today, I see wireless headphones for $6, t-shirts under $4, polarized sunglasses for $2, and a pair of 2TB thumb drives for $6. Wish takes a commission from merchants, and also gets paid by them for in-app ads and shipping.
The prices are absurdly low, because Wish is making certain concessions to get them that way. Most of the e-commerce world is obsessed with faster shipping, partially driven by Amazon. Wish tacitly concedes that it’s not going to win the battle for same-day shipping, and goes in the opposite direction: their average time from order to delivery was 22 days last quarter, a material improvement from 62 days in pandemic-disrupted Q2. Wish also has a relatively light touch on quality control issues, intellectual property, and other problems. There are 4,143 BBB complaints about their products, some of Wish’s offerings do not try especially hard to disguise their intended brand-name comp (lots of “legoe-compatible” toys), and it’s fairly easy to find euphemistic items like “car fuel filters” (i.e. suppressors for guns).
One viewpoint is that Wish is selling utter garbage. A more realistic one is that there is a large audience that’s willing to sacrifice quality, certainty, and delivery time because price is all that matters. A good ad hoc rule is that consumers satisfice on several criteria, and then maximize on one; airlines used to complain that they only offered basic economy seating because people who use price comparison sites always sort by lowest cost, and while Costco doesn’t give itself much margin flexibility, it does insist that its Kirkland brand must be 1% better on whatever trait matters most.
Wish’s target demographic is low-income. Their S-1 is full of references to the global middle class, and to households under under $18,000 a year. In a Forbes interview, the CEO says that buyers' credit cards are most likely to be declined just before payday.
So Wish is targeting the set of consumers who respond only to price. As it turns out, this is a large addressable market. It does make the Wish shopping experience more fraught, since users have to get used to parsing reviews to figure out which ones are fake, carefully reading product descriptions to ensure that they’re buying a phone rather than a case, or a brand-name toy rather than a set of LED display lights for the toy in question.
But this, too, is part of the model. Wish is rare among US e-commerce players in that it cites a monthly active user number. Not a count of paying users, but of total users of the app, regardless of whether or not they pay. As it turns out, Wish doesn’t just source its products from China; it gets its KPIs there, too: Alibaba, JD, and Meituan Dianping have all released this number, but in US e-commerce it’s relatively uncommon. Interestingly, one e-commerce player that did offer this number was Zulily, which is now privately held. It’s part of Qurate Media, parent company of QVC and HSN.
QVC and HSN pioneered a model of direct response advertising where the ads were just another form of content. It’s not as entertaining as other scripted shows, but the revenue per viewer-hour is higher, and if the ads are entertaining, the advertiser keeps the upside. Wish is thinking the same way: they claim that users spend an average of nine minutes a day on the app—low for a consumer entertainment product, exceptionally high for a product that’s literally one big ad. And within Wish’s commission-based ads is another ad layer: like Amazon and others, they’ve found that e-commerce search and product pages represent excellent inventory for ads, so they let sellers buy ads for their products on the site. As I’ve argued on Amazon, this is a way to price discriminate: it’s hard to get sellers to reveal their unit economics directly, but ad auctions show them automatically, so in-site ads are a way to charge every seller their expected profit. Wish plans to branch out from there; the S-1 alludes to the possibility that they’ll sell ads for external categories like travel and finance.
And where does this audience come from? Wish made $1.30bn in commission revenue in the first three months of the year. They spent $1.13bn on marketing in the same period, almost all of which was for ad buys. Like TikTok, they’ve paid up to acquire an audience that they can use to train their models so the next few million people they acquire are more profitable. As is increasingly traditional in S-1s, they offer a cohort analysis in terms of the gross profit per customer by year:
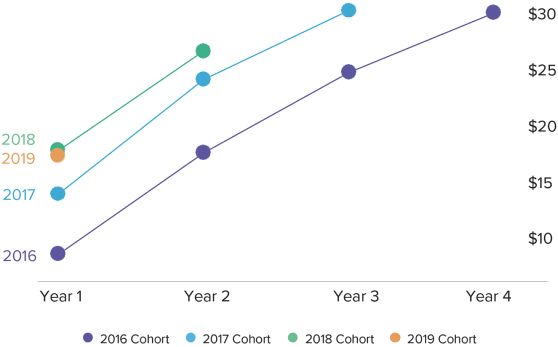
In one sense, Wish is an audience arbitrage: they find people who are browsing aimlessly on Facebook, and convince them to browse aimlessly on Wish instead. They slowly ratchet up how much they monetize those users, while also convincing merchants to spend more of their own gross margins running ads on Wish.
Wish’s very marginal customers and low-margin merchants create another interesting opportunity. Because Wish collects revenue upfront but remits it to merchants later on, the business actually generates some float. In a zero interest rate environment, that’s not an automatic moneymaker, but it does mean that Wish can and does use working capital lending (in the form of early payment to reliable merchants) and buy-now-pay-later financing as one more tool to keep merchants and customers on the platform. The same traits that make Wish’s customers and merchants less desirable to other e-commerce operators give Wish more ways to attract them through very low-level financial engineering.
It’s interesting to consider the long-term steady state of the business, which is currently halfway between TikTok and QVC. For some users, the Wish product just clicks; it’s entertainment shopping, with a low enough price point that the hit from gambling on dubious products isn’t so bad. But for a pickier audience, Wish is a terrible deal: taking four weeks to send customers a product that breaks right away is exactly what Amazon is trying to avoid. So Wish may end up churning through a large audience of not-quite-target customers, and retaining a smaller audience of diehard loyalists. This is not by any means a disaster. Qurate, for example, can handle a heavy debt load and return lots of capital to shareholders. Its audience isn’t huge, but at this point, they know exactly what they’re getting, and Qurate can reliably predict what they’ll spend.
Wish is the sort of company that will eventually get a catalyst-free writeup from a major short seller. The risk factors are basically a prompt for a diligent researcher. For example, Wish says it has 500,000+ merchants and that it frequently gets IP infringement complaints. All a short seller has to do is order some suspiciously affordable brand-name products, see which ones are obvious fakes, and include photos in their writeup. Or order some especially cheap off-brand products, and highlight the worst of the lot. Some short-selling due diligence is expensive, but the very nature of Wish’s product makes it cheap. The Luckin Coffee short report involved immense manual labor—reviewing receipts, lurking in stores, obtaining video footage of the business in action. A Wish short report can budget $100 or so for buying a grab bag of products (anyone planning such a report should factor shipping times into the expiration date of their put options).
The most helpful way to look at Wish is that it’s filling gaps. Slow shipping is cheaper than fast shipping, because the most lucrative customers are time-sensitive; low-quality products are damaging to most e-commerce companies' brand names, because they set high customer expectations. Even within Wish’s own revenue lines, there are examples of this: Wish offers one variant of its merchant ad product that promises rapid-but-expensive traffic instead of more gradual visits; they’ve replicated AWS’s spot-versus-reserved pricing, with the same price hit for immediacy. Like anyone else in the space, they’re making a fundamental bet that when they touch a large amount of economic activity, they’ll be able to detect where the margin opportunities are, and exploit them.
Elsewhere
Online-to-Offline
The WSJ rolls up some data on a trend I’ve cited off and on this year: big tech companies are buying more commercial real estate ($):
While Facebook, Microsoft and Google have said they would support working from home beyond the pandemic, that hasn’t appeared to have dulled their appetite for warehouses, data centers, retail stores and even more office space. This year alone, the five tech giants have expanded their real-estate footprint by more than a quarter, their fastest rate over the past decade.
At many tech companies, “dogfooding” is seen as an important part of the process. Yahoo couldn’t offer a search engine that Yahoo employees preferred over Google, while Microsoft employees use plenty of Outlook, Excel, and Powerpoint. But dogfooding can be done to excess—profiles of early Facebook point out that employees were generally not big users of Facebook, because playing with an addictive social product got in the way of building one. The real estate buildout is a reflection of the same phenomenon: online interactions are a superior substitute for many in-person ones, but the more talented employees are, the more valuable the bandwidth of in-person interaction is.
I wrote a bit more about the long-run steady state of working from home here in May.
<$10/month
In yesterday’s post, I mentioned the observation that there are very few viable subscription products that charge under $10/month. This makes every exception worth looking into: European neobank N26 now has a €4.90/month pricing tier. In this case, it looks like pandemic-induced price discrimination. This account tier is similar to the company’s core €9.90/month product, but without travel insurance, so presumably the plan is to get users at the current pricing and upsell them as soon as travel fully recovers. A bank is particularly well-positioned for this kind of upsell, since N26 can suggest an upgrade as soon as they start seeing transactions for hotels and plane tickets.
Movie Lots
Netflix is investing $1bn into a studio in New Mexico, helped along with some significant incentives from the state government. This looks like a story about California’s network effects unwinding, and that’s probably part of it—it’s easier to convince film workers to leave LA when they’ve already left—but part of it is due to cost pressure. Private equity firms have been buying up film lots ($, WSJ), and Netflix has always been very careful about controlling sources of cost inflation.
Taxes and Incentives
New York is considering raising parking fines and offering a cut to people who report illegally parked cars. Governments have been relatively slow to realize what consumer Internet companies figured out early: any labor-intensive surveillance activity can be carried out by, or at least significantly assisted by, a sufficiently large userbase. In most tech contexts, this means using a flagging/reporting function to a) triage moderation decisions, and b) train automatic moderation and spam-filtering features. But even absent training, it’s a useful technique. Since cities, especially large and expensive ones, will be in fiscal trouble for a long time post-pandemic, they’ll be likely to experiment with new revenue sources.
Unfairness
Tyler Cowen has a bullet-biting piece on why we should prefer unfair vaccine distribution until there are enough doses for everyone. The benefits of vaccination for a given population are nonlinear, in a Dickensian sense: 65% vaccination rate, R(t) of 0.99, result happiness. 64% vaccination rate, R(t) of 1.01, result misery.