Longreads
- Will Larson has a great piece on founding Uber's site reliability engineer organization. One measure of a startup's maturity is that early on, when they're moving fast, it's the SREs who make sure they don't break things. (A company is no longer a startup when the function with this responsibility is the legal department.) It's a good look at how companies organize and reorganize as they grow, and how they manage tradeoffs. Good line: "my manager would later confide that he had initially been concerned that I was going to walk in and immediately solve the problems he’d been struggling with. Of course, it would soon become clear that those very excellent plans worked best on paper."
- Institutional Investor profiles hedge fund manager Chris Hohn. Hohn is another case study in the arbitrageur to generalist pipeline, starting out with a focus on special situations and then moving on to focus on growth stocks. For any investor who's been in business for a long time and grown their assets under management, you'll tend to see style shifts, and it's possible that the ability to do this well is one difference between good analysts and good portfolio managers. There are, for example, plenty of analysts out there who are well-equipped to make the case that a company trading at 50x today's sales is really trading at just 20x 2027 adjusted EBITDA, but a fair number of those growth stories are partly contingent on other growth stories. (A company with 130% net dollar retention, for example, either needs to have customers who are all growing over 30% on average or will eventually swallow their economics and kill them off, and such growth rates are usually dependent on some outside funding.) So the magnitude of Hohn's switch is worth thinking about, not just the direction.
- Pearl Leff on the virtues of memorization. This is a good piece on the mechanics of how memorizing lots of facts helps you reason faster, since a) you're more likely to remember something if you can put it in context, and b) you're more likely to draw analogies to things you already know—or question them—if you know more. One way to think about this is that accumulated knowledge must be very valuable because workers' wages rise for most of their careers, even though the ability and willingness to work long hours tails off after a while, and fluid intelligence peaks early. This could be some kind of grand conspiracy to underpay twentysomethings, but that would be hard to maintain for very long. Experience isn't the only thing that matters, but it matters a lot, and this post is a good look at why.
- And on a related note, Dan Luu has thoughts on "Cocktail Party Ideas," specifically generalizations people make about a field from outside that field. It's a great sanity-check to ask: if this were a true generalization about someone else's work, why would everyone put up with it? And that goes both ways: jobs that look easy have subtle difficulties, but jobs that look very difficult may either have some clever tricks that simplify things or some subtle perks that make the effort more than worthwhile.
- Tom Critchlow asks: Why are executive dashboards so bad? This is a great question! At a single-product software company, the executive dashboard is probably the second most important piece of internally-developed software, and everywhere else it may well be first, and yet quality is uneven and low. As it turns out, this piece is yet again related to the question of how much seemingly-unnecessary detail you should worry about, and the answer is: a lot. If a manager isn't confident in which of their inputs lead to measurable outputs, then good outputs are a matter of luck.
Books
- The Machine that Changed the World: Is there available alpha in reading a book that was an MBA staple for a decade-plus? Probably! This book extolls the wonders of Japan's auto industry, particularly its efficiency and adaptability, and urges American (and even more so European) manufacturers to get on board. The ideas in the book, about managing inventory, moving fast, and listening to workers are well-known, if not always practiced. But it's still good to go back and look at the primary source material. One useful observation is that European manufacturers were, on average, even less efficient than American ones. That's notable because the very early history of imported cars gaining marketshare wasn't about Toyota and Nissan: it was Volkswagen, which sold small and trendy cars in the US a bit earlier. So the US auto industry's loss of domestic share wasn't a monocausal phenomenon (unless "they were badly-run" counts as a cause): at first, the kinds of vehicles they focused on mattered, but over time, the way they built them mattered more.
- Once Upon a Car: last week I read about the US auto industry's struggles in the 70s and 80s. This week, it was the 2000s. Some of the story is the same: high labor costs and a reliance on big, gas-guzzling vehicles. But one big difference is the nature of corporate drama. In the 70s and 80s, the office politics were largely internal: Lee Iacocca and Henry Ford II had a falling-out, so Iacocca went to Chrysler. But in the 2000s, the investment industry had grown and the auto industry had gotten comparatively smaller, so companies like GM and Chrysler were feasible targets for activist shareholders and corporate restructurings.
Open Thread
- Drop in any links to topics of interest to Diff readers.
- Coming attractions in the next few weeks include a look at Datadog, the leveraged loan market, and stories of success and failure in trying to transplant a new corporate culture. Readers with strong opinions on any of the above are invited to reach out.
- When fundraising is abundant, companies aim for growth at any cost. In the near future, it looks like it will be harder to get funded, but there's still a lot of dry powder for both private equity and venture. So what does the ideal strategy look like when it's still hypothetically possible to raise giant rounds, but not something you can count on?
A Word From Our Sponsors
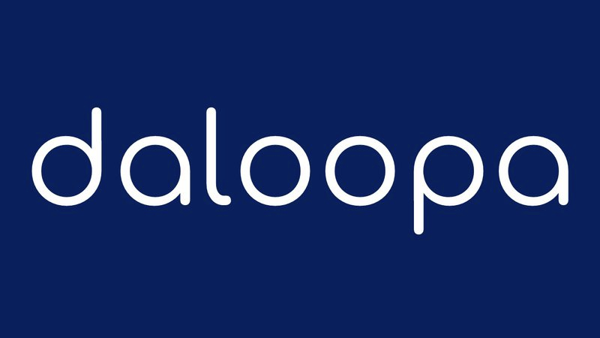
Here's a dirty secret: part of equity research consists of being one of the world's best-paid data-entry professionals. It's a pain—and a rite of passage—to build a financial model by painstakingly transcribing information from 10-Qs, 10-Ks, presentations, and transcripts. Or, at least, it was: Daloopa uses machine learning and human validation to automatically parse financial statements and other disclosures, creating a continuously-updated, detailed, and accurate model.
If you've ever fired up Excel at 8pm and realized you'll be doing ctrl-c alt-tab alt-e-es-v until well past midnight, you owe it to yourself to check this out.