In this issue:
- One Edge or Many? Reflections on Renaissance Technologies—It's fun to speculate on how Renaissance Technologies pulled off their long-term winning streak, but the nature of what they've built, and of how long they've been operating, means that it would be hard for anyone outside the company to replicate it. Rentec turns out to have evolved a very efficient system for creating proprietary strategies and keeping them proprietary.
- Retention—OpenAI's equity kerfuffle.
- Training Data—The data Slack wants, and the data they don't.
- Social Networking Primitives and Local Maxima—The latest from Meta's social network cloning vat.
- Renting—The case for never owning a home.
- Ads—Walmart's late-adopter edge.
This issue of The Diff is brought to you by Mercury.
One Edge or Many? Reflections on Renaissance Technologies
Over a thirty-year period, the Medallion Fund produced annualized gross returns of 66.1% with a 31.7% standard deviation, an unmatched but easily-misunderstood track record.[1] So, starting from the moment Renaissance started to get media attention in the early 2000s, the question has been: how did they pull it off?
Asking how they did it is a good question, because the existence of market inefficiencies should in some sense be confusing, and their persistence even more so. On the other hand, running a systematic investment firm with a persistently good track record over long periods is basically one long exercise in making "How did they do it?" a question for which you can't get any good answers. There are two driving forces for this:
- Compounding knowledge within the company
- An elegant but partly-accidental system for ensuring that this knowledge doesn't leak out.
You can treat quantitative finance as a hybrid between two fields: academic researchers have been looking at large datasets of long-term returns for a long time—the University of Chicago established the Center for Research in Security Prices in 1960, and Alfred Cowles had done it a generation earlier. On the practitioner side, Ben Graham was running a fairly systematic process early on, and William O'Neil did something similar with growth stocks. Michael Milken is an early example of cross-fertilization; he got interested in junk bonds because of academic research, then turned them into a real business.
Jim Simons started collecting voluminous financial data in the very early days of his investment career; The Man Who Solved the Market talks about him scouring datasets for patterns, hiring people to transcribe interest rate histories that hadn't been digitized, etc. Given how small quantitative finance was before the 1980s, it's possible that Renaissance has always had a data advantage compared to the rest of the investing world.
And that data advantage compounds, especially because working with messy data requires tacit knowledge: sometimes, the numbers are wrong, sometimes they're right-but-misleading, and sometimes the issue is subtle enough that you need lots of eyes on the data to see what's going on—one person notices suspiciously round numbers at suspiciously uniform timestamps, another looks at a chart or scatterplot and wonders why it's lopsided, someone else notices that a big jump on the chart coincides with some unpredictable event like an earthquake or pandemic, so a model that predicts it is probably overfitting.
One of the better efforts to figure out just what Renaissance was up to was a thread on the now-defunct Nuclear Phynance message board. A few pages are archived (e.g. here), but one comment that seems not to be archived said that the company once claimed that most of its strategies don't make money after transaction costs on a standalone basis, but that the combination of those strategies still pays.
That's a good recipe for both compounding returns to scale and a competitive moat. If there's some trade that costs 10 basis points in transaction costs for a round-trip transaction, and you have a signal that predicts 2bps of excess return, it's worthless. But once you have half a dozen such signals, you have something profitable, and each additional signal you add is closer to pure profit.
This interacts in a funny way with the general process of systematizing markets. Some of the early Renaissance strategies—early enough that people felt comfortable talking about them—sound like technical analysis with smarter jargon. Trend-following really did work in commodities and currencies for a while, and back when making a chart of the Krone actually required some degree of data sourcing and programming, being the first person to notice that you could draw lines on charts was a genuine source of alpha.
But what happens when more people follow that signal? Before, trending might have indicated that currencies under-react to macroeconomic events—perhaps the Danish central bank cut rates, and savers slowly realized that they'd make more money if they denominated savings in a different currency, but they didn't all act at once. That would create noticeable trends in the market, and when those trends petered out, it would indicate that the new equilibrium had been reached. But if the marginal Krone buyer was increasingly someone who had drawn lines on charts rather than a Dane trying to preserve their purchasing power, the trend would go on a bit longer, but be a bit faker, and the end result would be yet another iteration of the most important chart in finance: strategies start to get crowded, produce artificially good returns while that's happening, and then crash before reverting to a lower-return regime.
And in that lower-return regime, whoever is running the most signals in parallel for a given asset class is the most likely beneficiary, both because they'll capture more of the dollars of alpha available and because they're one principal component analysis away from measuring the contribution of that one element of the strategy.
Run this process again and again, at multiple timescales, in currencies, commodities, rates, and equities, and you can imagine systematic investors accumulating libraries of proprietary strategies that work for them but wouldn't work for anyone else.
And they won't just do that: they'll have their own vocabulary, their own infrastructure, their own coding standards—Rentec has been hiring programmers and implementing algorithms since the early 80s, and they've had very low turnover, so whatever tools they're using have had a lot of time to coevolve around their staff, the mental models they use to understand markets, and whatever nasty backwards compatibility problems arise when a live trading system has been continuously interacting with multiple complex markets for decades.
What someone on the outside might see is that there are a lot of financial research papers that fail to replicate in a good way: they spotted a genuine opportunity, and as soon as they published it got arbed away.[2] But what might be going on instead is that a signal had a half-life where it produced returns above transaction costs, and then an indefinite afterlife where it still added to a broader strategy even if its own returns were not worth the effort.
If that's the case, there's no point in asking for specifics about how Rentec makes their money. For one thing, they may have a completely different vocabulary for describing it, since they were building internally what other people discovered later, and since the initial assumptions you make color how you describe what you do next.[3] If they wanted to, Renaissance could probably give you a long list of strategies that make them money but wouldn't make you money. But if they did that, they'd still be giving up some of their returns—perhaps some competitor had assembled four separate 2bps edges in the 10bps-round-trip hypothetical asset class above, and two of the Rentec ideas were enough to flip that strategy from unprofitable to profitable. When that happens, there's now more competition in those tiny alphas, and suddenly returns aren't so great.
But there's still the question of why these ideas don't leak out. The Nuclear Phynance thread alludes to a competing fund offering large bounties for former Renaissance employees. (This is unsporting, especially when the premise is that you buy, say, $10m of alpha created by the firm's research process at the cost of a $1m sign-on bonus from someone who knows about, but didn't develop, that process. On the other hand, this model is a way to put pressure on an equally-lopsided approach of just not paying people very much of the value they create. The healthy steady state is one where each of these kinds of misbehavior are about equally common.) There aren't many examples of Rentec alumni spinning off to found other funds. A few of them run investment vehicles, but those seem to be places to deploy their Rentec profits since Medallion itself is at capacity. In fact, there are some CVs like Robert Frey's: get good at math, use that to get a job in finance, make a ton of money in finance—so you can retire from trading to focus on math again.
Other big systematic funds have produced sizable spinoffs: D.E. Shaw has Two Sigma, Magnetar was co-founded by a Citadel alum, and both Shaw and Citadel are at least nominally part of earlier quant lineages (Morgan Stanley's statistical arbitrage group and Princeton-Newport, respectively). [Edit: an earlier version of this post stated that D.E. Shaw came from Morgan Stanley's Process-Driven Trading group, but that group formed later. David Shaw did work at MS in the 1980s, doing statistical arbitrage, but not under the PDT name.] Not so for Renaissance!
They have had occasional defections, with some litigation, but much less so than other companies. And that's the other secret to their success: a firm that is maximally exploiting a set of strategies other firms don't have, in a way that's hard to copy, is a firm that is a very good place to keep your money for as long as they'll let you. More senior employees have more accumulated capital, and will own more of the fund but know more of the secrets. Newer employees are contributing, and probably under-earning relative to the value they add (but presumably earning much more over time than they'd get anywhere else). This creates a nice symbiosis, where the optimal setup is for retiring employees to keep capital at the firm and gradually get bought out by the people who still work there. As long as the pace of this buydown exceeds the decay in their general quant skills and their specific knowledge about Rentec's signals, it's in their aggregate interest to keep secrets.
You can think of the billions of dollars each year in anticorrelated alpha that the firm delivers as some combination of an incentive program for current employees to keep running those regressions and spotting those inefficiencies, and as a retrospective payoff for retired ones who might need a monetary reminder not to reminisce in much detail.
And this actually brings us back to the first point. Nobody compounded their money at 66% annualized for thirty years. Not Jim Simons, not James Ax, not Leonard Baum, not Elwyn Berlekamp.[4] Everyone gets cashed out to some degree, because it's economically implausible for their money to keep compounding that fast forever. And when a given strategy can produce high returns on capital based on the specialized knowledge of the people creating and implementing that strategy, the specialists will have all the leverage in deciding how returns will be allocated. What's the market price for a stream of returns that has equity-like volatility but more than 6x the returns, and that does best when equities drop? It's probably a fee structure that produces a lower return than the S&P for the same level of volatility, given that some of the volatility is driven by high returns in 2000 and 2007-8, which were tough years for equities, while returns were also higher in 1994 and 1998, which were difficult times for fixed income and emerging markets, respectively. The most sustainable structure for such an investment vehicle is for all of the returns to go to the people who are producing them, except for a slice that goes to people who could produce a subset of them but could be paid not to.
It's somewhat depressing to think that there's no economically rational explanation for you being able to invest in Medallion, to replicate Medallion, or to found a new Medallion. That is certainly a bummer. But there's a Platonic aspect to business advice: the object-level stuff is entirely too specific, and there's an advice-EMH theory where most of the time, the advice you're in a position to understand is the advice you came up with on your own. But Renaissance does have some replicable traits: even if we don't know what signals they used, we have some idea of how they found the right people—their founder came from math, which, along with philosophy, is one of the more broadly-applicable fields of academic endeavor. Instead of trying to evaluate people based on their financial knowledge, Renaissance focused on their academic skills. And it built an organization that was a great home for former professors or for people who veered off the professor track. “Find something to excel in, find a profitable analog to that, and recruit the people whose skills you can evaluate” is still a hard-to-replicate formula. But, unlike Renaissance, it'll probably happen more than once.
The easy-to-misunderstand part is that 1.66^30 doesn't reflect what anyone got from putting their money into the fund: Medallion started returning outside capital in 2002, finished in 2005, and capped its size at around $10bn in 2010, returning all profits to partners at the end of each year. If they'd actually compounded at 66% annually, the $20m in capital they had in 1988 would have grown to $80 trillion in thirty years. ↩︎
Though Simons has claimed that when Rentec backtests published papers, they don't find any signals that really work. ↩︎
For example, if you're doing a factor analysis to determine returns contributions or hedging, you're typically looking at how much a given factor explains an asset's returns, and then how much of what's left over is explained by something else. So the set of factors you find is going to be different depending on which you start with. ↩︎
It must have been a tough day at the office the first time Rentec hired someone whose name wasn't also part of the name of a famous theorem. ↩︎
A Word From Our Sponsors
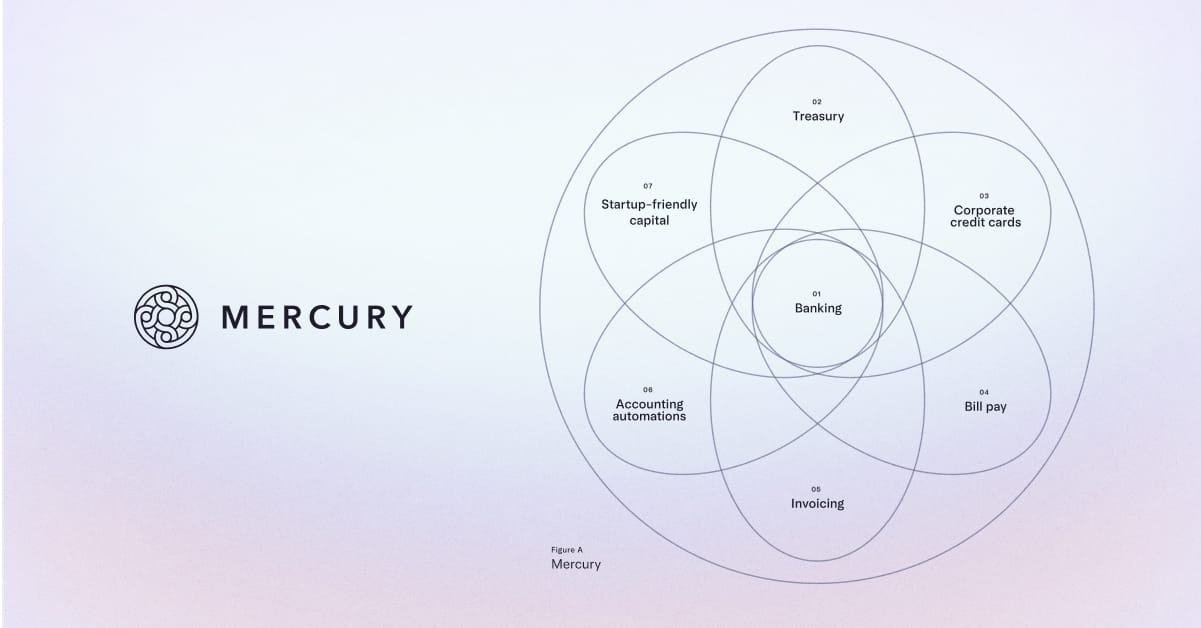
Power all your financial operations with Mercury
Financial operations are needlessly complex. Founders and finance teams have to cobble together a patchwork of tools that aren’t integrated with each other, costing valuable time, and leading to errors.
Mercury solves this broken system with banking* and software that powers all your critical financial workflows — all together within the one thing every business needs — a bank account. And with new bill pay and accounting capabilities, you can pay bills faster, stay in control of company spend, and speed up reconciliation.
Simplify your finances with Mercury and experience what it’s like to be more precise, have more control, and work faster than ever before.
*Mercury is a financial technology company, not a bank. Banking services provided by Choice Financial Group and Evolve Bank & Trust®; Members FDIC.
Elsewhere
Retention
High future investment returns and esprit de corps are not the only ways to improve employee retention. There's also OpenAI's approach of retroactively taking back equity if employees quit and refuse to sign a perpetual NDA. (After this story came out, OpenAI walked this back pretty aggressively, claiming that they will not take already-vested equity.) Training foundation models is a case where it's entirely plausible for one person to walk out the door with hundreds of millions of dollars of intellectual property, because every lab's list of tricks will be different and the rewards for having the best-performing model are so high.
This approach is extremely aggressive—it's standard to forfeit unvested stock and potentially some deferred compensation, but quite unusual to have prior pay clawed back, especially in an opaque way. From an AI risk standpoint, one thing to keep in mind is that there are many strategies that are popular because they work well in iterated games. But there's a different set of strategies that make more sense in a situation where, the next time you interact with someone, the world will be so different that whether or not you treated them fairly will be a minor detail.
Training Data
Slack turns out to be automatically opting users in to allowing their data to be used to train AI models. It is, to be fair, a great corpus of realistic conversational text, and thus a tempting source of data for a company that has a number of customer service-adjacent products. But it's also an expensive kind of data: first, there's the cost of some companies dropping their Slack usage because they don't want their proprietary information in an LLM, and second, there's the cost of making sure the LLM doesn't accidentally emit personally identifiable information. A more realistic use case is the one Slack itself cites: users need to know which channels to join, and will be more engaged if Slack is recommending the right emoji reactions. This kind of thing does require some kind of opt-in for data sharing, but it doesn't involve Slack training a model based on proprietary information and then somehow finding a way to get that information out before it shares something it shouldn't.
Social Networking Primitives and Local Maxima
Meta is once again testing a view-it-just-once photo product. They've done variations on this before: Stories stuck around, but Poke and Slingshot didn't. So far, this test is for internal use only, but at Meta's scale any such feature is implicitly a way to solicit feedback from reverse engineers, followed by tech blogs and Twitter.
One of the enduring debates in social media is over how many networks any one person needs. Like the Pirahã, the usual answer is one, two, or many: either one network is the central switchboard for all of the relationships you have that touch the digital world, or there's one for close friends and family and another for outward-facing things—the place to post anything that you're okay with strangers tying to your real name and job. Or there's one network for every use case, and the networks add and drop features based on these use cases. Meta's original bet was that one social network was what everyone needed, but they've since gotten a more nuanced view. The question now is whether there are clusters of behaviors that are so distinctive that there are multiple mutually-incompatible categories of networks, such that a feature that works for one will actually dissuade users from trying another.
Disclosure: long META.
Renting
The NYT has a good piece on people who rent indefinitely. Buying a house is, by design, a way to make a levered bet on an investment that tends to rise in value over time, and that produces ongoing income in the form of shelter. Over long periods, it's not a great asset class, and for individual investors it's even worse since buying a house means making an illiquid bet on the same local labor market that is, for most people, already their biggest un-hedged bet.
Ads
On Walmart's earnings call last week, they highlighted the fact that e-commerce and advertising have roughly 3x the company's overall margin, so when their customers move online, Walmart's earnings can rise even if overall revenue growth is anemic. The bigger a company's brick-and-mortar operation, the wider this margin gap can be: that retail revenue is also first-party transaction data that can be used to better target ads.
Diff Jobs
Companies in the Diff network are actively looking for talent. See a sampling of current open roles below:
- A company reinventing the way Americans build wealth for the long-run is looking for a legal ops hire; someone who can stand up entities and negotiate with third parties. JD preferred, but not required. (NYC)
- A fintech company using AI to craft new investment strategies seeks a portfolio management associate with 2+ years of experience in trading or operations for equities or crypto. This is a technical role—FIX proficiency required, as well as Python, C#, and SQL. (NYC)
- A top prop trading firm is looking for an intellectually curious, mathy generalist to work on projects spanning business strategy, technology, and markets. (NYC)
- A diversified prop trading firm with a uniquely collaborative team structure is looking for experienced traders and PMs. (Singapore or Austin, TX preferred)
- A startup building the world’s most performant parallel-EVM is looking for a low level engineer with C++/Rust/CUDA experience who wants to deploy their EVM core to web3 projects. (Remote, EU preferred)
Even if you don't see an exact match for your skills and interests right now, we're happy to talk early so we can let you know if a good opportunity comes up.
If you’re at a company that's looking for talent, we should talk! Diff Jobs works with companies across fintech, hard tech, consumer software, enterprise software, and other areas—any company where finding unusually effective people is a top priority.