In this issue:
- Price-First or Thesis-First Research?
- Aging Up
- Housing
- Virality
- LLM Arms Races
- The Uber of X
Today's issue of The Diff is brought to you by Dover, an applicant tracking system.
Price-First or Thesis-First Research?
If you're investing and believe that you have some kind of edge, the research process will always result in a trade, but there are two very different ways to get there. In general, people are either starting with an apparent mispricing and then building a thesis around it, or they're starting with a thesis and then using it to identify mispricings. You could just as easily say that investment research is about finding truths most people are wrong about, and that the two main processes are to start with people being wrong or to start by determining what's true. Think of it mistake-first or truth-first, or as Jane Austen versus science fiction: you're either starting out by paying very close attention to human behavior and how it goes wrong, or you're taking some external assumption seriously and then asking "as the world changes rapidly, in what new ways will permanent aspects of the human condition express themselves?"[1]
These general models apply across many different strategies and timescales. For example, a good fundamental example of a mistake-first thesis is from spinoffs. Companies will, not infrequently, hive off some unrelated part of their business as a separate company and distribute shares pro-rata to existing shareholders. Often, the company does this because the assets are hard to analyze together (GE is in the process of splitting into aerospace, healthcare, and energy equipment); sometimes it's because one asset is much less popular than another. An example of the latter is when mining company Anglo American spun off its coal operation, Thungela Resources, a few years ago. Coal was a small part of the business and a big part of their PR headaches, so the asset was actually more of a liability as part of a larger entity. Since Anglo American shareholders had all been complaining about owning a coal stock, they were happy to sell, even at a low price. The result is that in the two and a quarter years since Thungela became an independent company, its shares have returned over 1,000%.[2]
On the systematic side, a common source of alpha is taking the other side of a transaction where, for regulatory or incentive reasons, your counterparty will not trade in an economically rational way. The most benign instance of this is payment for order flow, which, looking past all the negative PR, is really about market makers paying brokers and their customers to get access to trades that they know are uninformed. And in this case, they're using a very narrow definition of "uninformed," simply meaning that any given trade is very unlikely to be a tiny piece of a much larger trade. Citadel Securities does not care if you're buying a few Nvidia call options because you have rock-solid evidence that next quarter's revenue beat will be even bigger than the last two—they will have hedged or sold by then. What they do care about is the risk that the first order they fill is from a large fund in the process of taking a hundred-million or billion-dollar position in Nvidia, because it means the flow of future trades will be nonrandom, and nonrandom in the wrong direction.[3]
Another systematic case of non-economic buyers is when index funds' membership changes. There are really two strategies here: predicting what will be added to or removed from the index, and trading ahead of index funds after these changes have been announced. These can both be sources of alpha, though sometimes it gets crowded and people lose money. A decent illustration of both of these features is to look at the stocks that were dropped from the S&P 600 small-cap index in the last index update. Some stocks move between indices, but the ones leaving the small-cap index are just seeing net sales from indexers, with no buyers. Looking at prices over the last month, the small-cap index itself was up slightly in the weeks before the index revision, but these stocks had dropped an average of 15%. The day the index revision press release hit, they were down an average of 9%. A quarterly catalyst predicting moves of that magnitude is worth paying attention to! There are many investors who are obligated to systematically buy or sell these stocks based on index changes, so an investor who can predict either changes in the index (ideally) or the resulting flows (in a less perfect world) will do well. It's not as if the index providers are dumb, or that losing a few basis points on stocks like Computer Programs and Systems or Netgear is a fair price to pay for not having to hire expensive investment professionals. It's just a necessary feature of index investing that leads to a large cohort of investors who can’t be too picky about the price, and trade on a short, predictable timeline.
Truth-first strategies try to produce alpha in a completely different way: ignoring what other people think (at first), and finding something that's true but that few people understand. A common area where this happens is navigating the supply chain to find the place where there's the least slack. The obvious example that comes to mind right now is starting with excitement about transformers, getting optimistic about cloud hyperscalers, and then getting deliriously bullish on Nvidia. And there's a long history here; when jet engines first started being used widely in civilian aircraft, the obvious bet was to invest in airlines—but, while they did well in the 60s, the big dividend was to travelers. The actual big money bet was that vacation destinations like Las Vegas would do extremely well in an environment where long-distance air travel was faster and cheaper, and that also turned out to be a more durable trade.
Systematic investors going truth-first are either finding a better theoretical way to value some asset or are finding a faster way to execute a known trading algorithm. Some kinds of trades are simple enough that the main differentiator is speed, while there are some kinds of trades whose value hinges on some question like "how does the correlation between X, Y, and Z change as prices get to more extreme levels?" These trades are betting against a misconception, in a way, but the misconception is either "it's impossible to do this any faster" or "the current models are good enough and the errors are random noise."[4]
Since these approaches are broad starting points that end in the same thing—executing a trade or implementing a strategy—they do end up overlapping. Someone who starts with an important fact about the world that isn't widely appreciated ultimately needs some theory for why it's not broadly appreciated: investors talk to management, analysts are always looking for stories, and the media like to cover big changes—so if there is some important shift in an industry's dynamics that will create winners or losers, betting on that shift with confidence requires having a view as to why so many people will get blindsided. It's not hard to find examples of this happening, of course, like Time Warner's CEO saying in 2011 that worrying about Netflix was "a little bit like, is the Albanian army going to take over the world?" or Blackberry's CEO saying in 2007 that the iPhone was "One more entrant into an already very busy space with lots of choice for consumers." But there are also plenty of examples of executives being dismissive but correct. Airbnb did affect the travel industry, but the hotel chains are still going strong; alternative energy matters, but the world still needs lots of oil and natural gas for a generation or two; crypto remains cool but is not a meaningful bear thesis for Visa or Mastercard at the moment.
Meanwhile, if you start with human error as the basis for your trading strategy, you have to worry about two things: first, that errors are not so erroneous after all, and second, that you're truly trading against an uninformed counterparty instead of betting against someone who is exploiting the same mistake more effectively than you. The US has the dominant share of actively managed assets in the world, and investors suffer from home-country bias where they allocate more of their capital than is reasonable to their country's markets. But US stocks have wildly outperformed the rest of the world over the last decade; so even if US investors were biased, they were lucky enough to be biased in the right direction. In the index rebalancing strategies discussed above, this repeated opportunity leads to competition to capture that alpha, so it's a Keynesian Beauty Contest where a growing component of the risk-adjusted returns to index inclusion trades comes, not from predicting what S&P Global will do or what Vanguard will do about that, but predicting what someone else might get wrong in their prediction.
Markets reward people who are right about something important that other people are wrong about. These two processes each hit two of the three: important and true, or important and misunderstood. But getting them right ultimately means nailing all three: finding something important that the rest of the world is wrong about, and understanding exactly why they're wrong—because that tells you what will change their mind and make asset prices show that you were right.
After all, every financial phenomenon is much older than one might think (a topic for future post!); a tech founder who is all-in on an important new product category, and who has to repeatedly raise money as operational problems, geopolitical issues, and a tough funding environment get in the way—that's a modern-sounding problem, but it's also a decent summary of Cyrus W. Fields and his efforts to build a transatlantic cable. ↩︎
Another reason companies do a spinoff is that they're pessimistic about the unit they're getting rid of, and simply don't want to be around for the ensuing disaster. Management is usually well-informed about their industry, but they're not infallible, and in this case they had no idea how much coal demand would stick around. But even aside from that, they spun off a company at a 2.7x EV/EBITDA multiple—low even for an industry in terminal decline. ↩︎
A market maker always runs this risk, because the business of accepting random buys and sells with a small spread between the buy and sell price is a very good one, so long as the buyers and sellers dribble in randomly. The spread they earn is basically an insurance premium against the risk that the next ten or twenty or fifty orders after the buy they take the other side of are also buy orders. One buy order can be causally connected to the next one, if a large order is broken up into many pieces, but a buy can only be causally connected to an immediate sell if somebody is running a buggy trading algorithm that is a price-taker on both sides of frequent trades. So the general source of excitement in that business is micro-scale bad news; good news, to a market-maker, is more amorphous, since it takes the form of higher volume coupled with higher volatility, meaning there are more buyers and sellers and they're less sensitive than usual to execution costs. ↩︎
Note that these two sketches of systematic advantages are at opposite ends of the speed/liquidity spectrum; one is shaving microseconds off the time it takes to do something, and the other is finding cases where 10-year-storm insurance is available at 100-year-storm prices or vice-versa. ↩︎
A Word From Our Sponsors
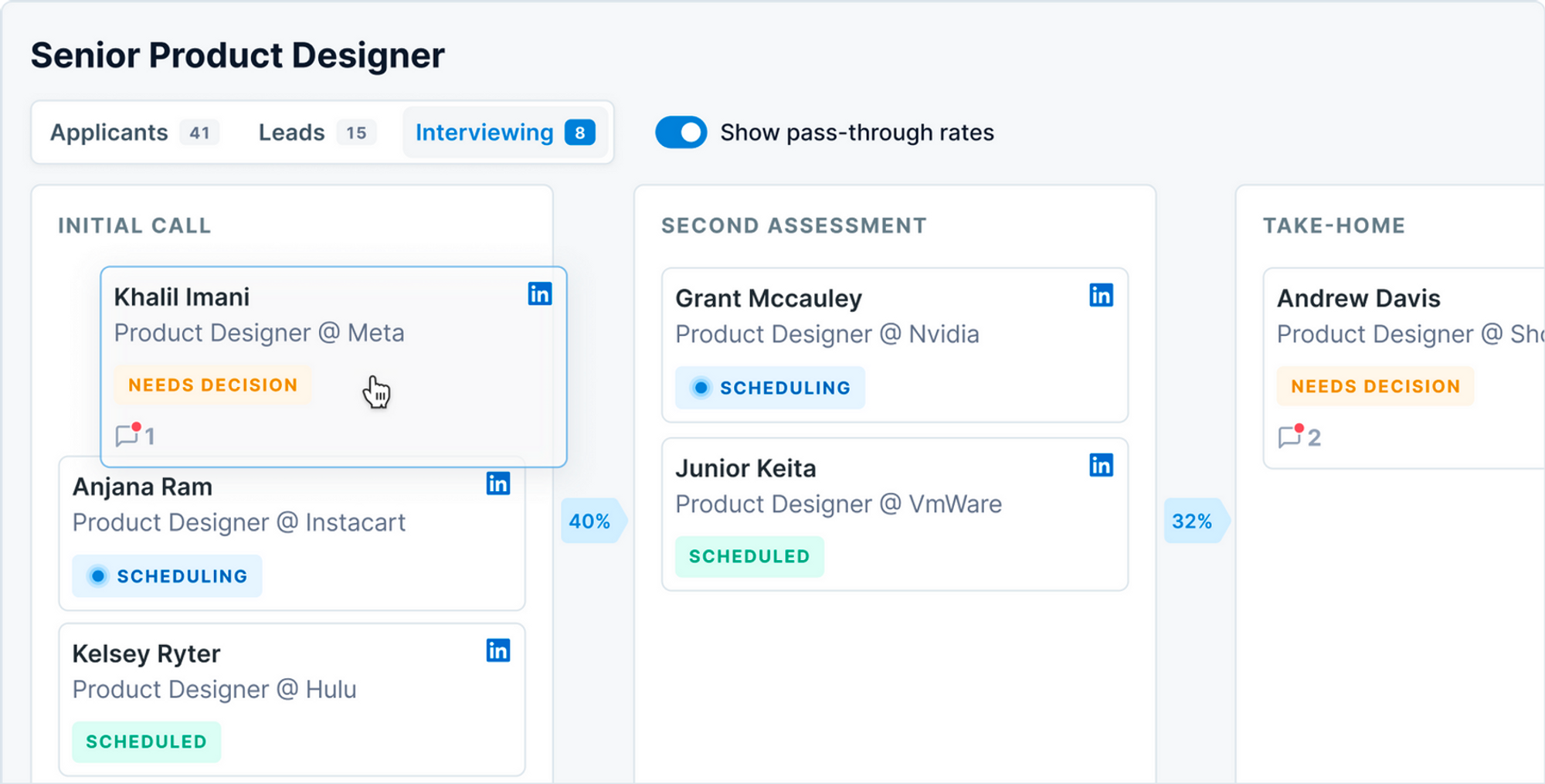
Dover is making world-class recruiting accessible to all.
Hiring the right talent is critical to any company’s success—especially for startups. Dover makes it easy for any company to run a world-class recruiting process, at no cost to the company.
Dover’s free ATS (Applicant Tracking System) is designed for founders and hiring managers to consolidate all their recruiting in one easy place. No more disorganization, dropped candidates, or chaotic scheduling emails.
Review applications at lightning speed, post to 100+ job boards (including free job posts on LinkedIn), send next steps to candidates in one click, create a beautiful careers page in seconds, and more.
Recruiting, simplified.
Elsewhere
Aging Up
In The Diff's writeup of Roblox ahead of their 2020 IPO, we looked at the company's strategy of aging along with its readers. If it can keep capturing the under-13 demographic, but also retain users for longer over time, its addressable market continuously expands. But this process is not easy, because it means continuously introducing features that are age-inappropriate for the demographic people associate the service with, even if they are reasonable given the service's actual and target users. So Roblox's CEO predicts that users will eventually meet, date, and form relationships through the site. He was careful to note that this is an age-gated set of features, and in a sense it's just an admission that as the site's users age while continuing to live in Roblox-owned digital spaces, some of their important life events will be mediated by Roblox. Other services have gone through this transition, and the real goal is to keep it from happening too fast.
Housing
A year ago, The Diff asked why German property developers are levering up so much ($). Since then, the three developers mentioned are down 10%, 13%, and 47%, while the DAX 30 German equity benchmark has returned 22%. One reason is, paradoxically, that it's gotten harder to build new homes in Germany, and it's getting more difficult over time ($, Economist). Usually, restrictions on housing supply are great for real estate speculators, especially if they're using leverage. (The typical levered real estate speculator does not think of themselves as such, but does worry more than average about neighborhood character, dangerous levels of shade, historical landmarks, and other issues that happen to raise rents and lower cap rates.) In Germany, real estate pricing is tightly regulated, so any given apartment is closer to a fixed-income asset than a risk asset. But refurbishing an apartment can reset allowable rents, and of course new properties can, too. By making it harder to do both, Germany has simultaneously induced a housing shortage and made owning real estate a worse business.
Virality
The usual cycle with social media sites is that they start out being very flexible and open, and are happy to have users even if those users are sharing content that sends other users off-site. But, over time, they try to get more content hosted on their own systems, and to promote it more relative to the competition. Google, while not a social platform, is the best exemplar of this: their early bet was that if they sent every searcher to the best possible result, searchers would keep coming back. But now location-based searches turn up Google Maps results, video searches bring up YouTube, travel searchers show Google Flights and Google Hotels, and everyone who relied on that traffic has to find a new model.
Twitter has historically had a high ratio of links-to-external-content to text, partly because it's a great medium for promoting an article with a viral headline, and partly because it's an exceptional tool for dunking. But Twitter has found that the five or ten minutes it might take to read a news story costs them a lot of ad views, while they have enough on-feed content to keep users entertained. So Twitter seems to have dialed down the virality of posts with links over posts with images and videos. This hasn't removed Twitter from the media discourse, of course, but it has a paradoxical effect: for a while, news coverage was downstream from Twitter, but if Twitter is hostile to long-form content, we'll see a bifurcation instead: Twitter discussions of complicated ideas will be downstream from the long-form sites that host thorough discussions of them, while the Twitter-native dialogue will be, as hard as this is to imagine, even less sophisticated and even more driven by reflex and partisanship.
LLM Arms Races
Meta plans to build a large language model, to be available next year, that will be more powerful than GPT-4 ($, WSJ). Like Llama 2, they're planning to open-source it. Meta's open source investments are an underrated aspect of their business: React and PyTorch both mean that Meta is a large user of something that many external developers are working to improve, and LLMs are no different—they can use them to enable different kinds of content consumption, like generating text summaries of videos for users who prefer reading over watching; by lowering the cost of human-level text, they increase the value of Meta's timeline ranking and spam-detecting technology. One other way to look at this is that Meta's making a somewhat unique bet among big AI players. The usual strategy among the biggest companies is to offer AI tools backed by their own hardware, outsourcing the exact use cases to end users. Meta is the biggest company to outsource the hardware used for inference to its end users, too. In a world where GPUs are in perennially short supply, that's exactly the wrong move, since it's lowering the price of something that isn't the limiting factor. But if hardware ordering ever overshoots, open source models will be the winners, as will the companies whose existing products are complements to them.
Disclosure: Long Meta, Microsoft.
The Uber of X
Many years ago I wrote a piece on how the Uber of X will be Uber: it's hard to beat scale advantages, especially when a given logistics network can carry more kinds of goods and can enable trips for more people. Uber has recently added code to their app for Taskrabbit/Angie-style "chores," like moving, cleaning, fixing appliances, etc.. As it turns out, Uber's network was less invincible than I had assumed in early 2019; Doordash was willing to spend enough to get the scale where it could pursue the same kinds of tiny optimizations that gave Uber an advantage. But the network and the customers remain valuable. For local services like this, one of the big costs is the dead time between jobs, and getting more people onto the same platform means better matching between workers and gigs.
Diff Jobs
Companies in the Diff network are actively looking for talent. A sampling of current open roles:
- A vertically integrated PE-backed cannabis company is looking for someone who has worked with complex operational and financial models in Excel. (Remote)
- A data consultancy is looking for a senior data scientist with prior experience in marketing data science and e-commerce—experience at Shopify would be ideal. (NYC)
- A new health startup that gives customers affordable access to preventative care and lifestyle interventions seeks a founding engineer. 7+ years of JavaScript experience is preferred (TypeScript is ideal), and payments experience is a plus. A great opportunity for anyone excited to make healthcare better by treating problems cost-effectively before they're catastrophic. (US, remote; Austin preferred)
- A proprietary trading firm is seeking systematic-oriented traders with ML experience—ideally someone who has displayed excellence in DS and ML, like a Kaggle Master. (Montreal)
- A company building ML-powered tools to accelerate developer productivity is looking for a mathematician. (Washington DC area)
Even if you don't see an exact match for your skills and interests right now, we're happy to talk early so we can let you know if a good opportunity comes up.
If you’re at a company that's looking for talent, we should talk! Diff Jobs works with companies across fintech, hard tech, consumer software, enterprise software, and other areas—any company where finding unusually effective people is a top priority.